Using Predictive Analytics Towards Effective Decision Making

What if you had the ability to foresee future business trends and make decisions with a high degree of confidence?
Predictive analytics, an advanced form of data analysis, offers this potential.
It combines historical data, machine learning, and statistical algorithms to forecast future outcomes, enabling you to make data-driven decisions with precision.
This powerful technology can transform various industries, from healthcare to marketing to finance, by uncovering trends, patterns, and anomalies in vast datasets that traditional methods might miss.
Predictive analytics moves beyond intuition and guesswork, providing real-world evidence to guide your decisions.
In a rapidly evolving business environment, the strategic use of predictive analytics can be a game-changer. It allows you to outpace competitors, enhance marketing efforts, streamline supply chains, and uncover new opportunities.
Curious about how it works? Read on to learn all about the transformative power of predictive analytics and discover how it can revolutionize your business strategies.
Fundamentals of Predictive Analytics
Predictive analytics is the process of using data, statistical algorithms, and machine learning techniques to identify the likelihood of future outcomes based on historical data.
By understanding the fundamentals of predictive analytics, including predictive models, historical data, and types of predictive analytics tools, you can make more informed decisions and improve business outcomes.
Understanding Predictive Models
Predictive models are statistical models used to predict future outcomes based on historical data. These models use a variety of techniques, including data mining, machine learning, neural networks, and decision trees.
Predictive models aim to identify patterns in historical data that can be used to predict future outcomes with a high degree of accuracy.
The Role of Historical Data
Historical data is a critical component of predictive analytics. By analyzing historical data, predictive analytics tools can identify patterns and trends that can be used to predict future outcomes.
Historical data can come from various sources, including customer, financial, and operational data.
Types of Predictive Analytics Tools
There are several types of predictive analytics tools available:
- Statistical tools: They analyze historical data and identify patterns and trends.
- Data analytics tools: These are used to analyze large volumes of data and identify patterns and trends.
- Machine learning: These tools build predictive models that can be used to predict future outcomes.
Applications in Various Industries
Predictive analytics is a versatile technology that has found applications in various industries. Here are some examples of how predictive analytics is used in different industries.
1. Predictive Analytics in Finance
Predictive analytics has revolutionized the finance industry. It's instrumental in fraud detection, risk management, and forecasting market trends.
Through thorough data analysis, predictive analytics empowers financial institutions to enhance profitability and make strategic decisions.
For instance, banks utilize it to preemptively identify customers at risk of loan default, enabling proactive interventions.
2. Optimizing Retail with Predictive Insights
Retailers are leveraging predictive analytics to streamline operations and enrich customer experiences. By scrutinizing consumer data, retailers gain valuable insights into preferences and behavior, enabling personalized marketing strategies and product offerings.
Predictive analytics aids in forecasting demand, optimizing inventory levels, and mitigating the risks of overstocking or understocking.
3. Healthcare and Predictive Analytics
In healthcare, predictive analytics plays a transformative role by facilitating early disease detection and prevention.
Analysis of patient data enables healthcare providers to identify individuals susceptible to specific ailments and intervene proactively.
For example, predictive analytics helps identify patients at risk of sepsis early, allowing for timely preventive measures.
4. Manufacturing and Supply Chain Predictions
Manufacturing sectors utilize predictive analytics to enhance production efficiency and streamline supply chain operations. Analysis of production data identifies bottlenecks and inefficiencies, driving process improvements.
Predictive analytics aids manufacturers in forecasting product demand and optimizing production schedules to meet market needs effectively.
Strategic Implementation
Developing a predictive analytics strategy and integrating predictive analytics into business processes are key steps in leveraging business analytics to make informed decisions, optimize operations, and tailor marketing strategies to specific customer segments.
By following these steps, businesses can stay ahead of the curve and gain a competitive advantage in today's data-driven world.
Developing a Predictive Analytics Strategy
A successful predictive analytics strategy begins with identifying business challenges that can be addressed using predictive insights.
It involves defining clear objectives for the analytics initiative and assembling a proficient team comprising data scientists, business analysts, and IT experts to execute the strategy. This team collaborates to extract actionable insights from data.
To formulate an impactful predictive analytics strategy, businesses must first pinpoint areas where predictive analytics can offer solutions. This may entail enhancing operational efficiency, making informed decisions, or tailoring marketing approaches to distinct customer segments.
Once the business challenges are identified, the objectives of the analytics initiative are delineated, spanning from forecasting future trends to mitigating risks and refining business processes.
Integrating Predictive Analytics into Business Processes
Predictive analytics integration into daily operations empowers decision-making. This integration can be achieved through real-time dashboards that provide insights, proactive alerts that flag potential issues, and incorporating predictive models into operational workflows.
To seamlessly embed predictive analytics into business processes, organizations identify pivotal decision points. These points are crucial for achieving business goals, such as optimizing inventory or enhancing customer satisfaction.
Predictive models are then developed to support decision-making at each of these junctures.
Challenges and Considerations
Implementing predictive analytics offers significant benefits for making data-driven decisions and anticipating future trends, but it also presents several challenges and considerations.
Managing Data Quality and Volume
Ensuring the accuracy and completeness of data is a major challenge. Predictive models rely heavily on the quality and volume of data.
Incomplete or erroneous data can lead to flawed predictions and potential risks. To address this, it is crucial to maintain accurate, complete, and current data for analytics.
Ethical and Privacy Concerns
Predictive analytics raises ethical and privacy issues, as these models often handle vast amounts of personal information.
It is essential to collect and analyze data in a lawful and ethical manner, adhering to all relevant privacy laws and regulations.
Transparency with customers about data usage and obtaining their consent for data collection is also critical to maintaining trust and compliance.
Staying Ahead of the Curve
Predictive analytics is a rapidly advancing field continuously developing new techniques and technologies.
It is vital to stay informed about the latest trends and innovations. This can be achieved by participating in industry conferences, networking with peers, and investing in ongoing education and professional development.
Conclusion
In today's rapidly evolving business world, making decisions based on data is crucial for maintaining a competitive advantage.
Predictive analytics has become a vital tool for organizations, enabling them to understand customer behavior, spot trends, and make strategic choices.
Utilizing predictive analytics allows businesses to streamline operations, enhance profitability, and secure a competitive position.
For example, retailers can use predictive models to anticipate demand, manage inventory efficiently, and refine pricing strategies. In healthcare, predictive analytics can identify patients at risk of chronic illnesses and facilitate targeted preventive measures.
To fully harness the benefits of predictive analytics, organizations must invest in appropriate technology, skilled personnel, and robust infrastructure. Ensuring data accuracy, relevance, and timeliness is essential.
As data volumes increase, it is imperative to adopt scalable and adaptable solutions capable of managing extensive datasets and sophisticated algorithms.
Overall, predictive analytics offers substantial advantages for organizations aiming to excel in today's data-centric business landscape.
By leveraging suitable technology, expertise, and infrastructure, businesses can unlock the potential of predictive analytics, driving growth and profitability.
If you're looking to stay ahead of the competition and find the top talent for your Netsuite needs, download Atticus Solutions' Salary Guide for valuable insights and recommendations.
Frequently Asked Questions
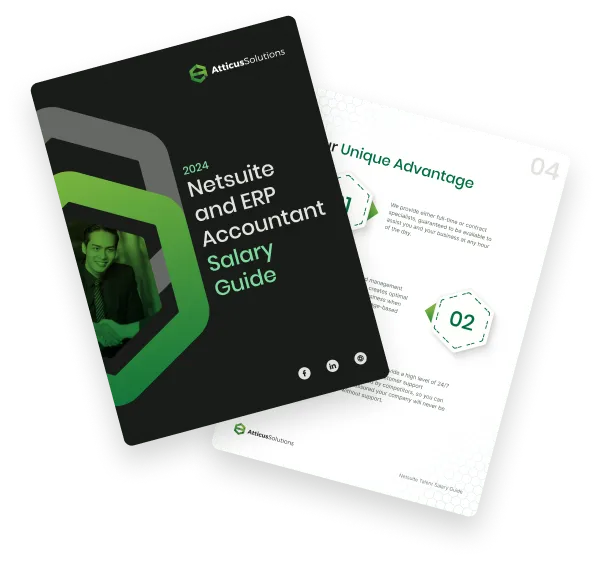
Compare NetSuite ERP talent salaries
Attracting top NetSuite talent with clear job descriptions is the first step. Understanding salaries is your next key move! Download this free salary guide to view talent costs, offshore hiring tips, and more
Learn how to scale your business

What is Your Strategy for Finding Rare Skill Sets like NetSuite Accountants?
Join our talented team. We are a small, passionate team with a commitment to hiring the best.
